Tech Focus: Language Models in Computer Vision
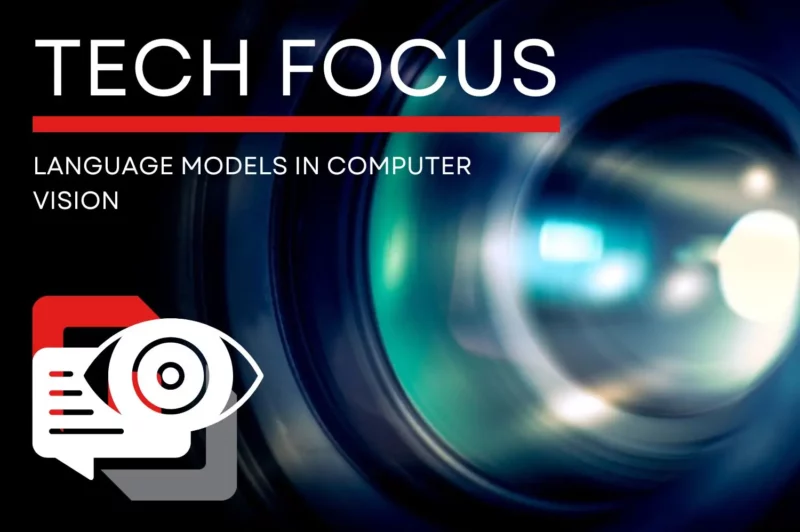
Language models are machine learning models designed for natural language processing tasks such as language generation. They can be used in computer vision applications to generate natural language prompts or structured queries for image-related tasks.
This Tech Focus will look at three types of models – Large Language Models (LLM), Small Language Models (SLM) and Vision Language Models (VLM).
Large Language Models (LLMs)
LLMs are designed to analyze, interpret and output data according to a huge amount of pre-learned information. Output is created using probabilistic methods to predict the next word or phrase, based on what it has learned during training.
All the big tech players have released LLMs, for example, Google’s Gemini, OpenAI’s GPT series, and Meta’s LlaMa. There are also a multitude of smaller companies with their own versions.
Azure AI Vision from Microsoft combines computer vision tools and LLMs. It is designed to analyze images and videos for applications such as image tagging, text extraction (OCR), and facial recognition. This makes it suitable for use cases in surveillance, automated inspection, and content management to name just a few.
Small Language Models (SLMs)
SLMs are built on the same concept as LLMs but trained on just a fraction of the data. While this may sound suboptimal, there are actually several advantages.
Smaller in size and complexity, SLMs have fewer parameters, making them less resource-intensive than larger models. By focusing on a smaller training library, SLMs are easier to fine-tune for very specific tasks or applications. This can result in more accurate and faster responses as less processing is required to obtain an outcome. Without needing access to the huge amounts of data required by an LLM, SLMs can be installed at the edge or offline devices.
In 2024, researchers at the Singapore University of Technology and Design created TinyLlama – an SML with 1.1 billion parameters, needing only 4.31 GB memory and running with 0.48s latency. This means running an SLM requires less computational space and power, resulting in lower cost for both training and deploying the model.
Being designed for just one application means that SLMs can deliver distinct advantages in healthcare applications. RadPhi-3 is one SLM which has been developed to summarize data from radiology reports. It is able to compare current and previous reports to identify changes, tag pathologies, and extract key sections from lengthy reports for faster evaluation. This supports radiologists and healthcare professionals working in remote areas, giving them immediate access to accurate data to help them make more informed decisions for improved patient outcomes.
Vision Language Models (VLMs)
VLMs (Vision-Language Models) have been developed to excel at processing both images and natural language text. These models need to understand both the input image and the text prompt, often using separate or fused encoders for vision and language. A vision encoder is trained on millions of image-text pairs, giving it the ability to associate images and text.
For example, DeepMind’s Flamingo VLM can describe images in a conversational manner and provide contextual information. It is suitable for various video analysis applications such as providing content description for accessibility purposes, or event detection in surveillance footage. As well as noting an anomaly in video, it is able to suggest what makes the event an anomaly, or what may have caused it in the first place. This VLM is highly effective at few-shot inference for vision-language tasks, meaning that it learns new tasks with very few examples. Flamingo claims to be capable of visual question answering (VQA) and open-ended reasoning.
Another example of a VLM is OpenAI’s CLIP (Contrastive Language–Image Pretraining). It uses contrastive learning to align image and text embeddings resulting in zero-shot classification – i.e. where the model performs a task it hasn’t been explicitly trained. It therefore learns to match images with text descriptions and classifies images without task-specific retraining. CLIP uses separate image and text encoders to process data independently, and is used in AI image generators such as DALL-E.
Using language models in computer vision applications
VLMs in particular offer exciting new opportunities for machine vision and computer vision applications. Image and video data collected by image sensors can be interpreted by the model and text cues can be generated to create detailed instructions. This might be used, for example, in logistics robots moving around a warehouse. Robots can be instructed to not only avoid collisions but also locate specific objects, move items from place to place or alert operators to low stock.
In medical imaging, the processing, analyzing and categorizing of scans and images could be vastly improved with the implementation of a VLM trained specifically for this task. There is already significant progress being made in areas such as radiology , and it’s likely that other fields of healthcare will be keen to adopt the power of VLMs.
With the ability to add contextual labels to image data, VLMs offer superior capabilities to industrial inspection and classification tasks. However, as with any learning tool, there are always risks! As The Register reported recently, if a model is trained on buggy code, it can be pretty hard to eradicate the bugs!
Want to know more about emerging technology? Sign up to our newsletter to keep up to date.